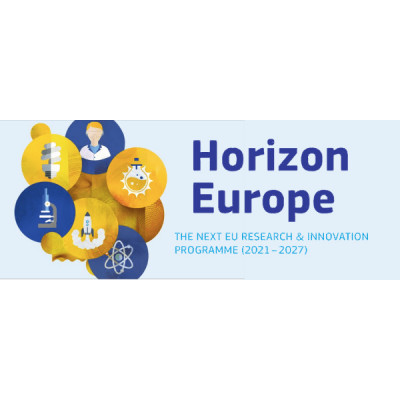
Understanding and Engineering Resistive Switching towards Robust Neuromorphic Systems: RobustNanoNet
Details
Description
Programme(s): HORIZON.1.1 - European Research Council (ERC)
Topic(s): ERC-2022-STG - ERC STARTING GRANTS
Call for proposal: ERC-2022-STG
Funding Scheme: HORIZON-ERC - HORIZON ERC Grants
Grant agreement ID: 101078843
Objective:
Resistive switching refers to the controlled change in resistance of an electronic material, e.g. metal oxide, via the creation and modulation of nanoscale filaments. Although its physics is not yet fully understood, resistive switching devices (called memristors) are promising as efficient artificial synapses in neuro-inspired computing systems. However practical challenges exist. Current devices excel in o nly a few of the performance metrics necessary for circuit and system integration. Moreover, they exhibit non-idealities causing neuromorphic systems using these devices to have low performance. The project will address this key issue by pursuing device-system co optimization across four objectives, aiming to engineer a single “hero” resistive switching technology with all the desired metrics. Aim 1 will develop resistive switching devices based on a new class of materials with broad compositional space, called high entropy oxides. Promising compositions will be fabricated in a high throughput fashion. In Aim 2, a proposed characterization method via a state-of-theart mid-infrared laser will help understand in-operando the filamentary switching at nanoscale and uncover the physical mechanisms behind its non-idealities. The fabrication and characterization will iteratively target a broad range of performance metrics. Some metrics can only be quantified across a population of devices, so Aim 3 will integrate the optimized devices on transistor circuitry for benchmarking at scale. Aim 4 targets the applicability of these devices to next generation neuromorphic systems for machine learning training. Preliminary work on a multilayer neural network validated this concept and indicated the need for cooptimization, as proposed. RobustNanoNet will address the interdisciplinary challenges towards a reliable resistive switching technology to support robust neuromorphic systems for energy efficient computing.